Stilling:
Stipendiat i datateknikk, signalbehandling eller kybernetikk
Søknadsfrist 7. oktober 2018
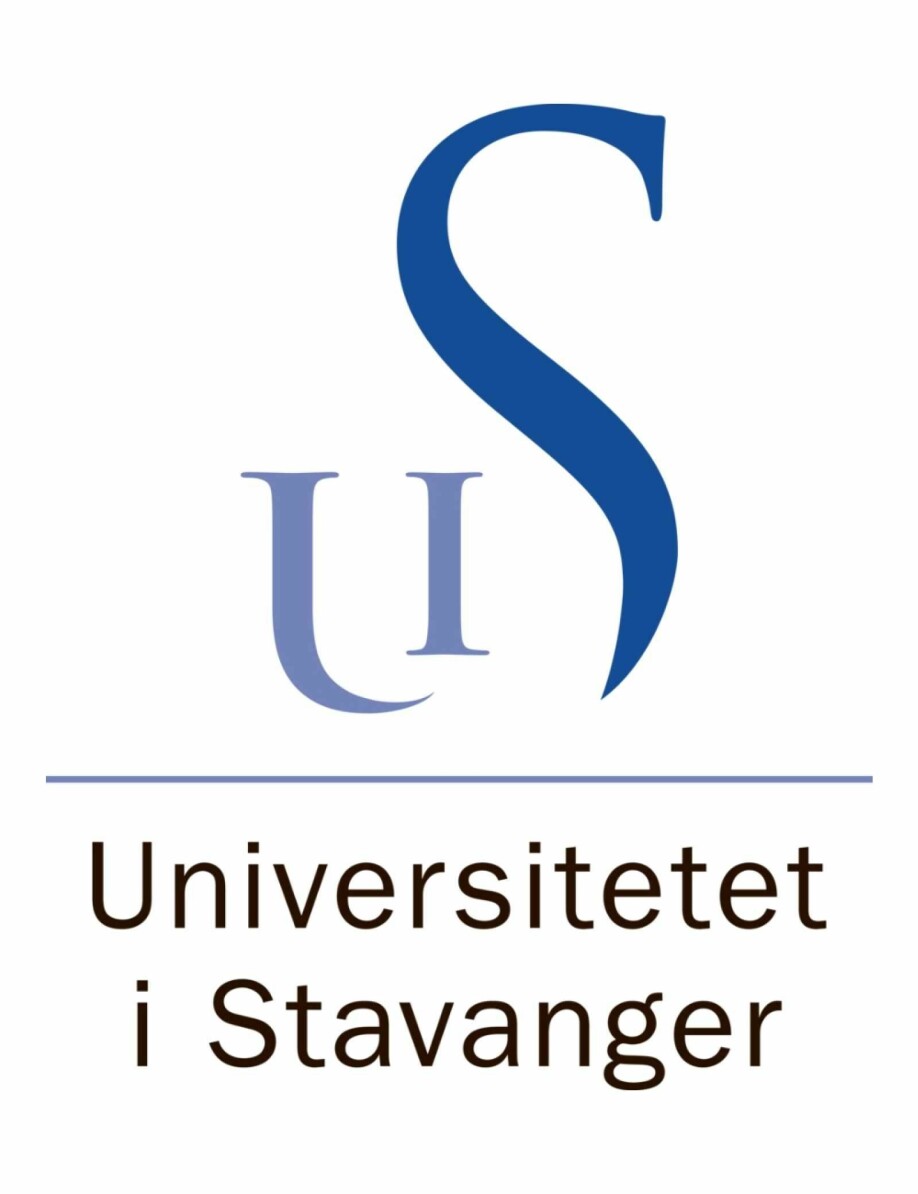
Universitetet i Stavanger har ledig stilling som stipendiat i datateknikk, signalbehandling eller kybernetikk ved Institutt for data- og elektroteknologi.
Dette er en utdanningsstilling som i hovedsak skal gi lovende forskere anledning til faglig utvikling. Stillingen har forskerutdanning fram til doktorgrad som mål.
Stipendiaten ansettes for en periode på tre år med ren forskerutdanning eller fire år med forskerutdanning og 25% pliktarbeid. Dette blir avklart i rekrutteringsprosessen. Stillingen er ledig fra oktober 2018.
Det er mulig å søke på opptil tre av følgende prosjekter:
- Orchestration and Control in Software-Defined 5G Radio Access and Core Networks
- Ultra-linear Digital-to-analogue Conversion
- Predictive analytics for personal monitoring devices (Heart rate and biomarkers)
- Big data analysis and data mining of geographically distributed time series data
- Distributed Deep learning for massive-scale graphs
- Scaling atomic multicast through content-based addressing
- Modeling and simulation of antimicrobial resistance in microbial communities
- Image analysis on computer tomographic (CT) perfusion images of acute stroke patients - Prediction of final stroke volume
- Conversational AI for information access and retrieval
- Consistency analysis of well surveillance data (Data mining)
Vennligst oppgi og ranger hvilke prosjekt du ønsker å jobbe med i søknaden din. For mer informasjon om hvert enkelt prosjekt, se nedenfor.
Søkere må ha en sterk faglig bakgrunn med femårig mastergrad, fortrinnsvis av nyere dato, eller tilsvarende utdanning som gir grunnlag for å gjennomføre en forskerutdanning. Karakter på masteroppgaven og veid gjennomsnittskarakter på masterstudiet må begge hver for seg tilsvare B eller bedre for å komme i betraktning.
Ved vurdering vil det bli lagt vekt på søkerens potensiale for forskning innenfor fagfeltet, samt vedkommendes personlige forutsetninger for å gjennomføre forskerutdanningen.
Den som ansettes må kunne arbeide selvstendig og i et fellesskap, være nytenkende og kreativ. Stipendiaten må ha gode ferdigheter i engelsk, både skriftlig og muntlig.
Stillingen anses som en viktig rekrutteringsstilling til vitenskapelig stilling ved universiteter og høgskoler.
Studiet gjennomføres i hovedsak ved Universitetet i Stavanger, bortsett fra et avtalt utenlandsopphold i et anerkjent relevant forskningsmiljø.
Stipendiaten lønnes etter Statens lønnsregulativ l.pl 17.515, kode 1017, kr 449.400 bto pr år. Stillingen gir automatisk medlemskap i Statens pensjonskasse som sikrer gode pensjonsrettigheter.
Prosjektbeskrivelse og nærmere opplysninger om stillingen fås ved henvendelse til:
- Instituttleder Tom Ryen, tlf 5183 2029, epost tom.ryen@uis.no
Opplysninger om ansettelsesprosessen fås ved henvendelse til Hr-rådgiver Janne Halseth, tlf 5183 3525, epost janne.halseth@uis.no
Universitetet har få kvinner i rekrutteringsstillinger innenfor fagområdet og oppfordrer derfor spesielt kvinner til å søke.
Søknaden registreres i et elektronisk skjema på jobbnorge.no. Relevant utdanning og erfaring skal registreres i skjemaet. Vitnemål, attester, publikasjonsliste og ev annen dokumentasjon som du ønsker det skal tas hensyn til, lastes opp som vedlegg til søknaden i separate filer. Dokumentasjonen må foreligger på et skandinavisk språk eller engelsk. Hvis vedleggene overskrider 30 MB til sammen må disse komprimeres før opplasting.
Prosjektbeskrivelser og kontaktpersoner:
1) Orchestration and Control in Software-Defined 5G Radio Access and Core Networks
The fifth generation (5G) of cellular networks aims to revolutionize the world of wireless communication. 5G will be characterized by ubiquitous connectivity, extremely low latency, and very high-speed data transfer. These characteristics will enable the use of 5G in a very broad set of application scenarios: from pervasive video to high user mobility; from broadband access everywhere to lifeline communications; from massive Internet of Things to broadcast-like services; from tactile Internet to ultra-reliable communications. For enabling this variety of applications, ambitious improvements with respect to 4G are needed: 10-100 times more connected devices; 1000 times higher mobile data volume per area; 10-100 times higher data rate; 1 ms latency; 99.999% reliability; 10 times less energy consumption; 5 times less network management operation expenses.For achieving the challenging objectives of 5G, the research community has been working on the Software-Defined 5G Radio Access and Core Networks, which extensively use virtualisation and softwarisation technologies, such as Network Function Virtualisation (NFV) and Software-defined Networking (SDN), in order to efficiently, flexibly, and scalably provide 5G network services. NFV and SDN will allow the profitable coordination of the heterogeneous radio network technologies, which include multi Radio Access Technology (multi-RAT) and multi-tier architecture (composed by macro-cells, small-cells, and relays), and device-to-device (D2D) communication, and can enable the Network Slicing. Network Slicing will allow the sharing of the same infrastructure for providing heterogeneous services, i.e. enhanced Mobile Broadband (eMBB), ultra-Reliable Low-Latency Communication (uRLLC), and massive Machine-Type Communication (mMTC).
The candidate will be working on approaches for the orchestration and control of the 5G radio and core network resources with different objectives, such as energy efficiency, Quality of Service (QoS), economical cost reduction, and dependability. The work can be focussed on algorithmic or experimental aspects depending on the will and skills of the candidate.
Knowledge of telecommunication networking is required, experience with optimisation and resource allocation or with Software-defined Radio (SDR) is useful but not required.
Supervisors: Associate professor Gianfranco Nencioni, gianfranco.nencioni@uis.no, and Professor Bjarne E. Helvik (NTNU)
2) Ultra-linear Digital-to-analogue Conversion
You will be joining a project that aims to use control theory to develop methods for digital-to-analogue conversion (DAC), enabling a dynamic range better than 1 part-per-million (equivalent to 21 effective number of bits), at high speed and with low latency. This level of performance has never been achieved before and will define the new state-of-the-art in the field. A semiconductor device with such capabilities will be key to enabling technology in several areas of industry and science as it will allow mass-market availability of unprecedented precision enabling techniques, which was not previously possible due to signal noise and distortion. In addition, it will dramatically improve the performance in any device already reliant on high-resolution digital-to-analogue conversion.
Today, analogue-digital conversion is ubiquitous. Analogue-digital conversion devices sold for USD 3.5 billion in 2017, and that market is increasing 10-15% annually. Better linearity, and thus higher resolution, will benefit systems using analogue-digital conversion. It is a key element in systems used in science, industry, medicine and consumer goods. High-resolution digital-to-analogue conversion and control has a wide array of applications, including: adaptive optics; semiconductor lithography, fabrication and inspection; laser interferometry; metrology (measurement science); imaging and manipulation in microbiology; chemistry and materials science; as well as scanning probe microscopy in general. Furthermore, methods relating to analogue-digital conversion will have major impact in renewable energy production and distribution, medical imaging and communications.
The best performing digital-to-analogue converter (DAC) available today achieves a resolution of 47 parts-per-million, or 15 effective number of bits. The project supervisor has already set the new state-of-the-art by building a DAC with a resolution more than 12 parts-per-million (17 effective number of bits).
You will be building on these results and taking it further! The work is multidisciplinary, drawing on control theory, electronics and signal processing, though the main tool will be model predictive control (MPC). You should therefore have a good background or strong interest in control theory, optimisation and estimation (Kalman or particle filtering). The work can focus on experimental results in the lab or be more oriented towards theory, depending on your background and interests. The project involves international collaboration with researchers from The University of Newcastle (Australia), Aalborg University (Denmark), SINTEF and the Norwegian University of Science and Technology. You will be expected to work in one or more of these locations for up to 1 year.
Supervisors: Associate professor Arnfinn A. Eielsen, arnfinn.a.eielsen@uis.no, Professor Andrew John Fleming (The University of Newcastle, Australia.)
3) Predictive analytics for personal monitoring devices (Heart rate and biomarkers)
Physical inactivity is a major challenge to global health and the problem is increasing rapidly: >5 million deaths each year are attributable to insufficient physical activity. Norway is among the European countries with the lowest level of spontaneous physical activity. Recent technological advances in personal heart rate and activity monitors in form of smart watches may make these devices potentially important tools for individualized guidance on physical activity. Despite the rapidly increasing use of smart watches there is yet no long-term documentation for their benefit and their potential role as diagnostic tools has not been established.
Characteristics of heart rate and changes in heart rate during exercise and rest, are strong predictors of cardiovascular prognosis. At the same time in the recent years, smart watches with integrated HR monitors for the first time became truly available to the average consumer. This makes meaningful research possible. Smart watches produce significant amounts of data, what calls for automated data analysis and requires application of big data tools in addition to a mix of other data science concepts such as machine learning and time series analysis.
The research will be performed primarily based on data obtained during the NEEDED 2014 and NEEDED 2018 study, containing heart rate (HR), power (W), ECG, blood samples, and other data for over 60 subjects collected during “Nordsjørittet”. Additionally, a mechanistic study was be performed during the spring of 2018 (NEEDED 2018), adding vast amounts of data on the relationship between heart rate, direct work measurement (powermeters), 12-lead ECG, and a large number of biomarkers, both during standardized physiological tests and during a bicycle race.
We aim to develop a model that predicts the expected, normal HR response to physical exercise in relation to biomarkers. This algorithm for the detection of a pathological heart rate response will be tested in future studies. A variety of methods will be explored ranging from basic feature engineering and classification, though time series analysis, to deep learning.
Supervisors: Associate Professor Tomasz Wiktorski, tomasz.wiktorski@uis.no, Professor Trygve Eftestøl and Professor II Stein Ørn.
4) Big data analysis and data mining of geographically distributed time series data
The main idea behind this project is to use spatio-temporal analysis and spatio-temporal data mining to detect slow-onset disasters. A slow-onset disaster is a disaster that does not occurs suddenly but instead happens gradually, and there is hope of detecting it early. One example of a slow-onset disaster is an epidemic.
During an outbreak of influenza or other disease, the behaviour of people change. For example, people may drive less or drive at other times than normal. Currently, the Norwegian Institute of Public Health (NIPH) detects an outbreak based on reported diagnoses from doctors. The doctors report this every other week, which means that there is a two-week delay from people starting to get sick to the NIPH knowing about it.
There is increasing monitoring in modern societies, and thus increased amounts of data that might be used to detect disease outbreaks earlier. The idea is to look for changes in how people behave, and particularly those changes that might indicate people being sick. One source of data that might be used for this is the number of cars that pass by automated road tollbooths or checkpoints from the road authorities that count the number of cars. A data set consisting of the number of cars passing a number of such checkpoints each hour exists and may be used for this purpose, with each checkpoint having a geographical position and associated time series. The flow of traffic is best measured by combining several time series at geographically distinct points in the same city. In order to find interesting patterns of behaviour change, data from multiple sources likely has to be considered. Other examples of data sources would be ticket purchases from public transport systems, utility usage in residential and commercial areas and purchases of medications.
This project will involve using state of the art data mining methods for spatio-temporal data mining and mining time series data. The project will also involve using machine-learning methods on graphs and spatial time series data.
The general research questions are as follows:
- RQ1: How to best store and analyse geographical time series data. Develop algorithms for analyse big geographical time series data.
- RQ2: How to combine data from the analysis of multiple sources from RQ1 in order to detect interesting changes in behaviour that might indicate a slow-onset disaster that is on its way.
- RQ3: How to best visualize the results from the analysis from RQ1.
This project is a collaboration between the Department of Computer Science and the Centre for Risk Management and Societal Safety (SEROS). This project deals with the technical challenges in such a project while there is an ongoing project at SEROS dealing with the societal safety issues.
Supervisors: Associate Professor Erlend Tøssebro, erlend.tossebro@uis.no, and Associate Professor Vinay Jayarama Setty.
5) Distributed Deep learning for massive-scale graphs
Graphs are ubiquitous data-structures and are used to represent social networks, knowledge graphs and biological networks. Traditionally many graph structural features are used for graph tasks such as community detection, link prediction, node classification, label propagation and recommendation systems. Recently application of network embeddings in the place of simple graph features has been increasingly popular for such graph mining. Techniques such as DeepWalk and Node2Vec have been proposed to learn network features vectors to solve these tasks in an unsupervised and generic manner. While these techniques are simple, their genericness renders them ineffective for specific tasks. Even though these techniques are promising for some applications, they have two major limitations: (1) they are based on shallow networks to learn neighborhood features, (2) they cannot scale beyond medium sized graphs.
Motivated by the above issues, in this project we seek to solve three main research questions: (1) Using a generic framework can we learn task-specific representations? (2) Can we learn richer representations to capture the hierarchical structures of the networks using recent advances in deep learning rather than simple random walks in the networks? (3) Can we scale the learning process using techniques such as distribution preserving sampling and asynchronous training? This thesis will address these research questions and propose a novel framework for learning task-specific, deep network embeddings for large-scale graphs.
To address these limitations, recent concept of Graph Convolutional Networks (GCNs) were proposed. However, scaling them to massive graphs requires distributed setting. This project sets design distributed deep learning technique to distribute and parallelize model training across several GPUs. There are challenges regarding partitioning the graphs and the aggregation of local models are aggregated using parallel paradigms in an efficient way.
The candidate is required to have a strong background in machine learning or deep learning and graph mining.
Supervisors: Associate Professor Vinay Setty UiS, vinay.j.setty@uis.no and Professor Krisztian Balog.
6) Scaling atomic multicast through content-based addressing
Atomic multicast is a fundamental building block for scalable distributed systems. Atomic multicast allows processes to reliably and consistently send messages to one or more groups of servers. A typical use case is a client broadcasting an update for a distributed object to a group of servers replicating that object. Atomic multicast allows updates to be applied consistently to multiple objects replicated by different groups. However, updates spanning multiple groups remain a challenge to the scalability of these systems.
Existing systems try to mitigate this cost by adapting the mapping of objects to groups to minimise the number of groups that process an update. This results in a complex and highly dynamic mapping of objects to groups.
Content-based addressing schemes, e.g. publish-subscribe, decouple senders and receivers in a messaging system.
The aim of this project is to investigate how content-based addressing schemes can be applied to further improve the scalability of atomic multicast systems, e.g. relieving clients and servers from the need to maintain complete address tables.
A special challenge is how content-based addressing can simplify dynamism in the assignment of distributed objects to groups of servers while maintaining correctness criteria.
Applicants should have a good understanding and experience building distributed systems.
Supervisors: Associate Professor Leander Nikolaus Jehl, leander.jehl@uis.no, and Professor Hein Meling.
7) Modeling and simulation of antimicrobial resistance in microbial communities
This project aims to develop mathematical models to better understand and predict how antimicrobial resistance spreads in microbial communities. Of particular interest is the spread of carriers for antimicrobial resistance (genes) in wastewater treatment plants since such plants are nodal points for further spread of into the environment. Two approaches will be examined in this project. The first is to use deterministic ordinary differential equations. The plant, bacterial populations and resistance genes are treated as continuous concentration state variables, and production and degradation are modeled by pseudo-kinetics and conversion stoichiometries. The second is individual-based and stochastic. An individual based model (IBM) is one where bacterial classes (guilds and genotypes), genetic carriers (plasmids) and viruses (bacteriophages) are treated as individual and discrete populations.
The work in this project will be conducted in collaboration with PhD candidates working on experimental studies on the spreading and ultimate fate of antimicrobial genes in a laboratory scale wastewater treatment system. Experimental data will be used for systems identification and calibration/validation of the proposed model. The candidate will join a group who also work on a closely related EU-funded project under the Joint Programme Initiative on Antimicrobial Resistance (JPI-AMR) with collaboration from top international groups at Lund University (Sweden) and Statens Serum Institute, Copenhagen (Denmark).
Applicants must have a strong academic background with master’s degree in dynamical systems, mathematical modelling, control theory/engineering (kybernetikk), or other related fields. A background in biology is not required, necessary courses in biology will be offered.
Supervisors: Associate professor Kristian Thorsen, kristian.thorsen@uis.no and associate professor Roald Kommedal.
8) Image analysis on computer tomographic (CT) perfusion images of acute stroke patients - Prediction of final stroke volume
Motivation: In Norway, 15 000 persons suffer from acute cerebral stroke annually. Acute cerebral stroke is the leading cause of adult long term severe disability, the leading cause for admission to nursing homes, and the third leading cause of death in adults in Norway [1]. Cerebral stroke is a common disease which has an enormous negative impact on the quality of life for the patients and a mortality of up to 25% in the acute phase [2], additional costs of health care in the acute and chronic phase are enormous for the society [1]. At Stavanger University Hospital (SUS) patients are routinely investigated using perfusion CT, and parametric colour coded maps describing the blood perfusion of the stroke area are calculated. These maps aid in the decision on who needs immediate thrombolytic treatment and are important in saving lives and reducing the possibility of severe disability. These parametric maps are far from perfect in diagnostic accuracy and further improvement of the methods in use is needed [3]. More accurate evaluation of perfusion CT may lead to better guidance of thrombolytic therapy and thereby better treatment of the patients.
The objective of the current project is to characterize the properties of tissue affected by stroke by utilizing advanced image processing and machine learning methods. It is especially important to be able to discriminate between infarcted tissue (irreversibly damaged tissue), hypoperfused tissue at risk for being irreversible damaged if not better perfusion is restored fast, and healthy tissue, since the relative sizes of these tissue classes have a major impact on patient life and probability of disability.
Methods: The supervision team has long experience in medical image analysis and the use of machine learning techniques. Classical image processing and analysis in terms of automatic segmentation, shape and size characterization as well as texture analysis of risk areas will be performed to extract relevant features from the images. Such features can be used as input to machine learning systems, classifying tissue areas. In addition, artificial intelligence (AI) in terms of deep learning neural networks will be explored. Deep learning neural networks have had a tremendous success in later years, providing state of the art results in many computer vision and image analysis applications. The use of autoencoder in deep nets provides a method for training nets for unlabeled or sparsely labeled data, which can be necessary for the dataset at hand. Also deep nets can provide a method for identifying the regions of an image that are important in terms of discriminating between patient classes, or tissue classes.
Dataset: All images needed for analysis are already collected making the feasibility very high. The available data set from SUS includes more than 1000 cerebral stroke subjects with completed perfusion CTs and a secondary CT or MRI after therapy. An additional note to the ongoing thrombectomy study protocol is needed to the Regional etic committee, but no new REK application. An important advantage of the available data is that the cohort is population based serving a homogeneous patient group from only one hospital in the entire region.
References: [1] Helsedirektoratet. Nasjonal retningslinje for behandling og rehabilitering ved hjerneslag. Oslo: Helsedirektoratet; 2010. 196 s, [2] Saumya H Mittal, Deepak Goel. Mortality in ischemic stroke score: A predictive score of mortality for acute ischemic stroke. 2017;3(1):29-34. [3] Y.W. Lui, E.R. Tang, A.M. Allmendinger and V. Spektor. Evaluation of CT Perfusion in the Setting of Cerebral Ischemia: Patterns and Pitfalls. AJNR 2010;31 (9):1552-1563.
Supervisors: Professor Kjersti Engan (UiS), kjersti.engan@uis.no and Professor and medical doctor Kathinka D. Kurz (SUS, UiS), Kathinka.dehli.kurz@sus.no
9) Conversational AI for information access and retrieval
Intelligent personal assistants and chatbots (such as Siri, Cortana, the Google Assistant, and Amazon Alexa) are being used increasingly more for different purposes, including information access and retrieval. These conversational agents differ from traditional search engines in several important ways. They enable more naturalistic human-like interactions, where search becomes a dialog between the user and the machine. Unlike in traditional search engines, where a user-issued query is answered with a search result page, conversational agents can respond in a variety of ways, for example, asking questions back to the user for clarification.
The successful candidate will work on the design, development, and evaluation of conversational search systems. In particular, the candidate is expected to employ and develop deep learning techniques for understanding natural language requests and generating appropriate responses.
The candidate is required to have a background in machine learning or information retrieval.
Supervisor: Professor Krisztian Balog, krisztian.balog@uis.no, and Associate Professor Vinay Setty.
10) Consistency analysis of well surveillance data (Data mining)
In practice of well surveys, petroleum field operators often get different well measurements in a form of non-synchronized time series with data of different frequency and quality. These measurements are often related to each-other and gaps in the data consistency may be filled in. As an example, the most of new oil and gas wells are now operated with installation of Permanent Downhole Gauges (PDGs) measuring in real time pressure and temperature inside the wellbore near the sand face (production intervals). The well pressure dynamics is governed by changes of well flow rates, which are often measured at surface, in some cases for a well template, not a single well. This causes inconsistency of well pressure and rate measurements, which may include absence of the measurements for some continuous periods of time (data gaps). This inconsistency should be eliminated or, at least, mitigated before analyzing well performance and updating reservoir simulations. Consistency analysis of such datasets may be focused on eliminating or reducing the inconsistency combining physical models and data mining approaches. Here, pattern recognition may be applied in combination with simple models of well inflow performance binding pressure and rate data in a joint ‘based-on-physics artificial intelligence’ manner. Resulted consistent data sets may be further analyzed in an automated mode to monitor well-reservoir parameters and well performance.
The work will be based on previous work focused on analysis and testing of existing possibilities to solve the problem described above in a combination with testing of new approaches. A ‘feature extraction’ approach was suggested and tested on simplified data sets. Further development and testing of the approach on more realistic data sets is the main objective. Further development of the ‘feature extraction’ approach to be applied to well surveillance data (time series of well measurements, which may be related to each other). The work will mainly focus (but not limited) on pressure-rate data sets. Testing the approach on different data sets: mainly synthetic well data resulted from reservoir simulations and probably real well data. Development and coding in Python of algorithms implementing the ‘feature extraction’ approach in application to the data sets described above.
Supervisors: Professor Chunming Rong, chunming.rong@uis.no, and Anton Shchipanov (NORCE)